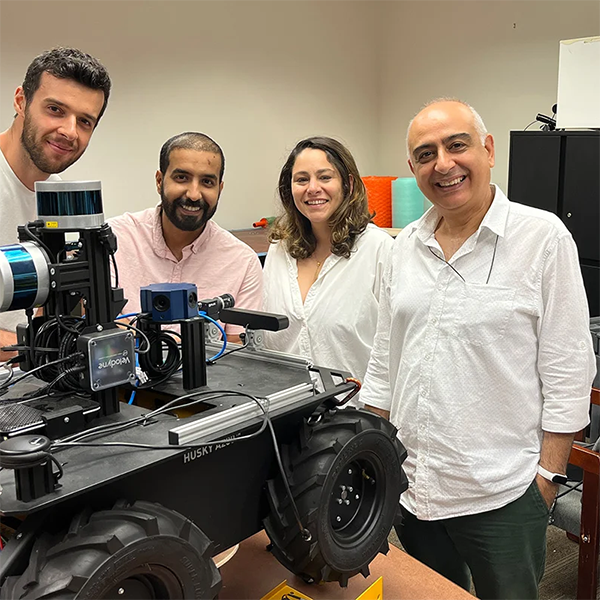
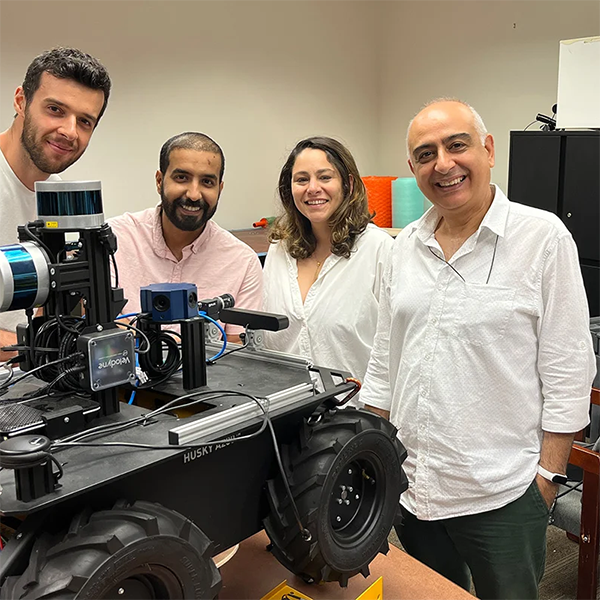
Research Terms
Society for Experimental Mechanics Civil Structural Testing Technical Division, Chairperson; 2011 - present
ASCE Structural Engineering Institute Structural Identification Committee, Chairperson; 2010 - present
ASCE Structural Engineering Institute Safety of Bridges Committee, Chairperson; 2009 - 2010
ASCE Structural Engineering Institute Technical Council, Secretary; 2009 - present
IABMAS Bridge Health Monitoring Committee, Secretary; 2005 - present
Researchers at the University of Central Florida have developed a method for reducing the time and cost of inspecting civil structures like bridges and buildings. Conventional methods for visually assessing civil infrastructures use subjectivity and may require long inspection time and high labor costs. Although some technologies (such as robotic techniques, augmented reality, and mixed reality interfaces) can collect objective, quantified data, an inspector's expertise is still critical in many instances. Such technologies, however, are not designed to work interactively with a human inspector.
In contrast, the UCF Collective Intelligence Framework uniquely blends AI with mixed reality (MR) and can be integrated into an MR-supported see-through headset or a hand-held device. An inspector can analyze damage in real time and calculate or assess its condition without performing any manual measurements. At any step of the analysis/assessment, the inspector can intervene and correct the operations of the AI. Another advantage of the system is that the inspector can analyze defects in a remote location safely while reducing the need for access equipment. For example, if the defect location is hard to reach and poses a safety concern (like under a bridge or atop high-rise buildings), the headset can zoom in and still perform an assessment without needing equipment like a ladder. Consequently, the methods can reduce inspection time and labor costs while ensuring a quantified, reliable, and objective infrastructure evaluation with human-verified results. The methodology is expandable for many types of structures.
Technical Details
The UCF invention integrates unique AI detection and segmentation algorithms into an MR framework that performs automatic detection and segmentation of defect regions using real-time deep learning operations. In infrastructure assessment, creating a large image dataset for machine learning is essential but can be challenging. As a solution, the invention offers an advanced data augmentation technique that enables the framework to generate a synthetically sufficient number of images (like cracks and spalls) from available image data.
In an example application, an inspector assesses a concrete pier using an MR headset integrated with the framework. While the inspector performs routine tasks, the AI system in the headset continuously guides the inspector and shows possible defects in real time. Once the inspector confirms a defect location, the AI system starts analyzing it by first executing defect segmentation, then characterization to determine the specific type of defect. If the defect boundaries need corrections or the segmentation requires fine-tuning, the inspector can make the necessary adjustments. The system uses the alterations made by the inspector to retrain the AI model so that the AI's accuracy improves over time.
Partnering Opportunity
The research team is looking for partners to develop the technology further for commercialization.
Stage of Development
Prototype available.
The University of Central Florida invention describes a comprehensive system for structural health monitoring at a global level using computer vision. The system integrates different algorithms and methods to develop a structure-oriented, computer vision-based structural response monitoring framework. It provides a guide for different structures in different application scenarios to use different types of algorithms and methods. The invention also proposes novel methods of using computer vision to conduct vibration serviceability, load distribution estimation, load rating, extraction of unit influence line for structural identification, and weigh-in-motion for structural evaluation. It can eliminate the issues and limitations in conventional structural health monitoring and condition assessment, such as a large amount of time, cost, labor forces to deal with the instrumentation and cable wiring, and possible traffic closure in bridge monitoring and inspection cases.
The University of Central Florida invention describes deep-learning-based generative model methods—Generative Adversarial Networks (GAN)—for civil structural health monitoring and damage prediction. Damage diagnostic data is limited, as data collection can be costly and challenging or because there are not enough data from damaged areas to train detection models. Using information collected from multiple structures, synthetic data samples can be generated by state-of-the-art GAN models and used to train damage diagnostic systems. These tools could aid engineers, stakeholders, and decision-makers (1) to perform diagnostics accurately and effectively on raw acceleration signals and (2) to be proactive rather than reactive in managing the life cycle of structures.
Partnering Opportunity
The research team is seeking partners for licensing and/or research collaboration.
Stage of Development
Prototype available.
Generative Adversarial Networks for Data Generation in Structural Health Monitoring, Frontiers in Built Environment, 11 February 2022, https://doi.org/10.3389/fbuil.2022.816644.
The University of Central Florida invention is related to a novel method and technology to aid structural health monitoring (SHM) experts and civil structure inspectors. The method uses the analyzed structure incorporated with virtual reality (VR) and augmented reality (AR) platforms to minimize or eliminate the need for site visits. The invention can enable a more efficient decision-making process with damage detection and load carrying information.
Partnering Opportunity
The research team is seeking partners for licensing and/or research collaboration.
Stage of Development
Prototype available.
Structural Health Monitoring of a Foot Bridge in Virtual Reality Environment, ICSI 2021 The 4th International Conference on Structural Integrity, Procedia Structural Integrity, Volume 37, 2022, Pages 65-72. https://doi.org/10.1016/j.prostr.2022.01.060